We test both bottom-up and top-down approaches in learning the phonemic status of the sounds of English and Japanese. We used large corpora of spontaneous speech to provide the learner with an input that models both the linguistic properties and statistical regularities of each language. We found both approaches to help discriminate between allophonic and phonemic contrasts with a high degree of accuracy, although top-down cues proved to be effective only on an interesting subset of the data
Research
This approach is essential to improve the ecological validity of our scientific theories and to facilitate their translation into practical tools that support better language learning outcomes.
My team and I investigate the key elements that shape how children learn language: sensory input, cognitive mechanisms, and social interactions.
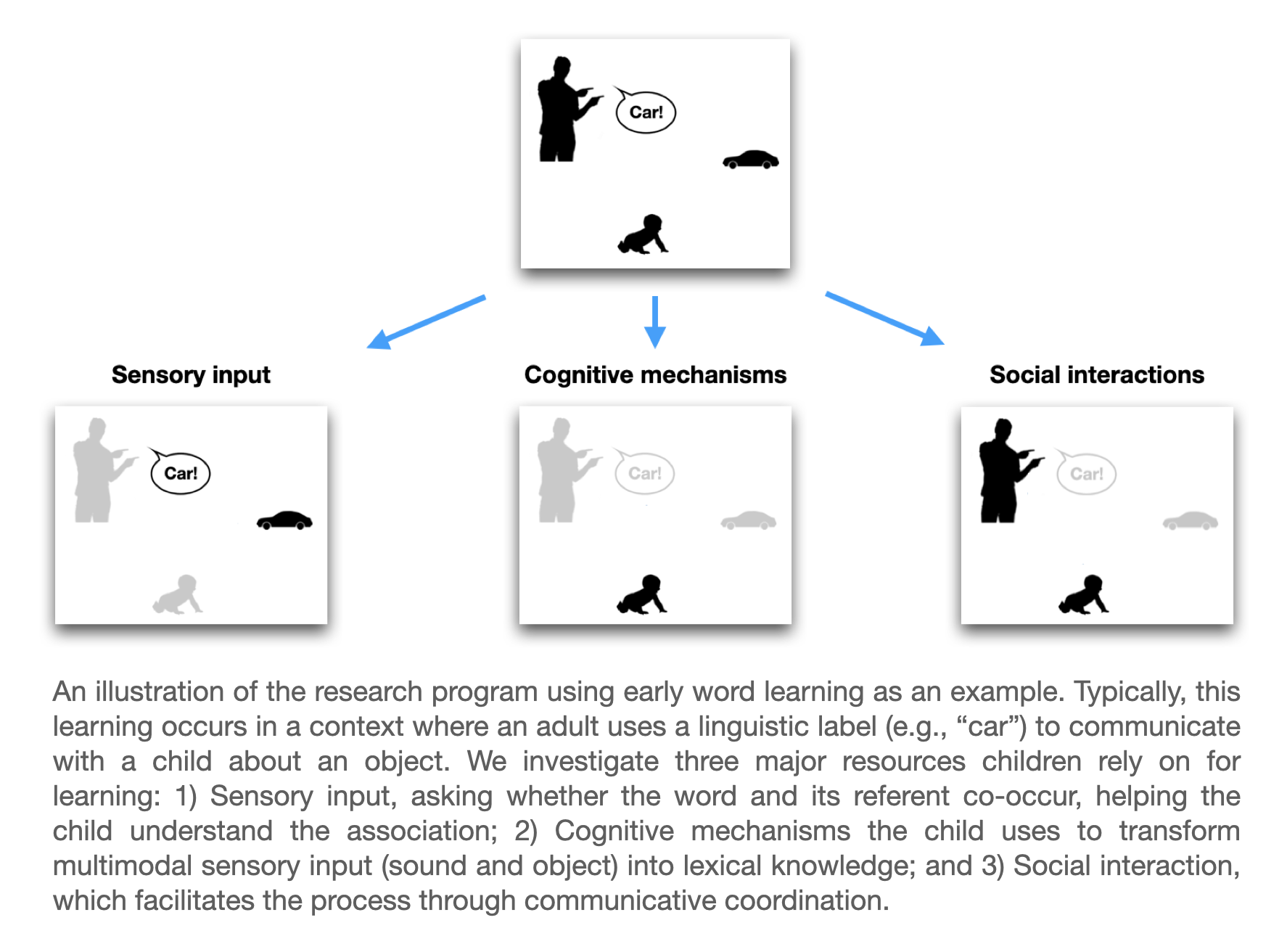
Sensory input
Language development starts with the sensory experiences of children—the words they hear and the things they see. We collect and analyze data from these experiences to understand how their richness influences learning.
One line of inquiry is exploring how children learn linguistic structures like sounds and grammar from natural speech. We specifically focus on how they manage to overcome the variability and noise to form stable mental representations:
-
Fourtassi, A., Schatz, T., Varadarajan, B., & Dupoux, E. (2014). Exploring the Relative Role of Bottom-Up and Top-Down information in Phoneme Learning. Proceedings of the Annual Meeting of the Association of Computational Linguistics (ACL).
-
Jiang, H., Frank, M. C., Kulkarni, V., & Fourtassi, A. (2022). Exploring patterns of stability and change in caregivers’ word usage across early childhood. Cognitive Science, 46(7).
-
Abstract
The linguistic input children receive across early childhood plays a crucial role in shaping their knowledge about the world. To study this input, researchers have begun applying distributional semantic models to large corpora of child-directed speech, extracting various patterns of word use/co-occurrence. Previous work using these models has not measured how these patterns may change throughout development, however. In this work, we leverage NLP methods that were originally developed to study historical language change to compare caregivers’ use of words when talking to younger vs. older children. Some words’ usage changed more than others’; this variability could be predicted based on the word’s properties at both the individual and category level. These findings suggest that caregivers’ changing patterns of word use may play a role in scaffolding children’s acquisition of conceptual structure in early development.
Cognitive mechanisms
While rich sensory input is crucial, children's cognitive abilities determine how effectively this input is transformed into linguistic knowledge.
We study the cognitive mechanisms children use to process sensory input, such as their ability to combine information from different modalities (e.g., mapping sounds to meaning). Using cognitive modeling, we mathematically characterize these skills and make quantitative predictions about learning. These predictions are tested both in the lab and in natural settings:
-
Fourtassi, A., Regan, S., & Frank, M. C. (2021). Continuous developmental change explains discontinuities in word learning. Developmental Science, 24(2).
-
Abstract
Cognitive development is often characterized in terms of discontinuities, but these discontinuities can sometimes be apparent rather than actual and can arise from continuous developmental change. To explore this idea, we use as a case study the finding by Stager and Werker (1997) that children’s early ability to distinguish similar sounds does not automatically translate into word learning skills. Early explanations proposed that children may not be able to encode subtle phonetic contrasts when learning novel word meanings, thus suggesting a discontinuous/stage-like pattern of development. However, later work has revealed (e.g., through using more precise testing methods) that children do encode such contrasts, thus favoring a continuous pattern of development. Here, we propose a probabilistic model that represents word knowledge in a graded fashion and characterizes developmental change as improvement in the precision of this graded knowledge. Our model explained previous findings in the literature and provided a new prediction – the referents’ visual similarity modulates word learning accuracy. The models’ predictions were corroborated by human data collected from both preschool children and adults. The broader impact of this work is to show that computational models, such as ours, can help us explore the extent to which episodes of cognitive development that are typically thought of as discontinuities may emerge from simpler, continuous mechanisms.
We also investigate how language is refined over months and years of development. Our methods allow us to study the complex interactions between how knowledge is organized in a child’s long-term memory (e.g., the lexical network) and the characteristics of their natural learning environment:
-
Fourtassi, A., Bian, Y., & Frank, M. C. (2020). The growth of children’s semantic and phonological networks: Insight from 10 languages. Cognitive Science, 44(7), e12847.
-
Abstract
Children tend to produce words earlier when they are connected to a variety of other words along the phonological and semantic dimensions. Though these semantic and phonological connectivity effects have been extensively documented, little is known about their underlying developmental mechanism. One possibility is that learning is driven by lexical network growth where highly connected words in the child’s early lexicon enable learning of similar words. Another possibility is that learning is driven by highly connected words in the external learning environment, instead of highly connected words in the early internal lexicon. The present study tests both scenarios systematically in both the phonological and semantic domains across 10 languages. We show that phonological and semantic connectivity in the learning environment drives growth in both production- and comprehension-based vocabularies, even controlling for word frequency and length. This pattern of findings suggests a word learning process where children harness their statistical learning abilities to detect and learn highly connected words in the learning environment.
Social interactions
The transformation of sensory input into linguistic knowledge is facilitated by social interactions. We study early interactions between children and caregivers to understand how and when communicative skills emerge and how these skills specifically support language acquisition.
Automated tools enable us to analyze these interactions on a large scale as they naturally occur in children's environments. This approach offers valuable insights into how children communicate spontaneously, learning to take turns and coordinate shared meanings:
-
Agrawal, A., Nikolaus, M., Favre, B., & Fourtassi, A. (2024). Automatic Coding of Contingency in Child-Caregiver Conversations. Proceedings of the Joint International Conference on Computational Linguistics, Language Resources, and Evaluation (LREC-COLING).
-
Abstract
One of the most important communicative skills children have to learn is to engage in meaningful conversations with people around them. At the heart of this learning lies the mastery of contingency, i.e., the ability to contribute to an ongoing exchange in a relevant fashion (e.g., by staying on topic). Current research on this question relies on the manual annotation of a small sample of children, which limits our ability to draw general conclusions about development. Here, we propose to mitigate the limitations of manual labor by relying on automatic tools for contingency judgment in children’s early natural interactions with caregivers. Drawing inspiration from the field of dialogue systems evaluation, we built and compared several automatic classifiers. We found that a Transformer-based pre-trained language model – when fine-tuned on a relatively small set of data we annotated manually (around 3,500 turns) – provided the best predictions. We used this model to automatically annotate, new and large-scale data, almost two orders of magnitude larger than our fine-tuning set. It was able to replicate existing results and generate new data-driven hypotheses. The broad impact of the work is to provide resources that can help the language development community study communicative development at scale, leading to more robust theories.
-
Nikolaus, M., & Fourtassi, A. (2023). Communicative Feedback in Language Acquisition. New Ideas in Psychology, 69.
-
Abstract
Children start to communicate and use language in social interactions from a very young age. This allows them to experiment with their developing linguistic knowledge and receive valuable feedback from their – often more knowledgeable – interlocutors. While research in language acquisition has focused a great deal on children’s ability to learn from the linguistic input or social cues, very little work has investigated the nature and role of communicative feedback, a process that results from children and caregivers trying to coordinate mutual understanding. In this work, we draw on insights from theories of communicative coordination to formalize a mechanism for language acquisition: We argue that children can improve their linguistic knowledge in conversation by leveraging explicit or implicit signals of communication success or failure. This new formalization provides a common framework for several lines of research in child development that have been pursued separately. Further, it points towards several gaps in the literature that, we believe, should be addressed in future research in order to achieve a more complete understanding of language acquisition within and through social interaction.
In conclusion, we leverage modern Machine Learning to develop theories of language development that can be tested and refined in real-world settings. This ecological approach ensures that our findings are both scientifically robust and more directly applicable. For instance, it can guide the design of educational tools and therapeutic interventions grounded in theory, which, by confronting other real-world challenges, provide critical insights for further refining those theories.